Introduction
In a rapidly evolving AI landscape, the proliferation of regulatory frameworks to guide the responsible development and deployment of AI has become both a necessity and a challenge. While this growing body of work reflects a global commitment to addressing the ethical, legal and societal implications of AI, it also presents risks, including regulatory duplication and overgeneralization. Organizations face the complexity of selecting frameworks that align with their unique needs and strategy, balancing compliance and innovation.
At Naaia, we are committed to staying at the forefront of AI regulatory compliance. By integrating regulations from key regions such as the European Union, the USA and China into our solution, we have established a solid foundation for AI risk and compliance management. Our latest integrations of the ISO/IEC 42001, NIST AI RMF and LNE Process Certification for AI frameworks further strengthen our ability to meet the specific needs of our customers and the wider AI industry. In this article, we will explore each framework individually, then provide a comparative analysis to highlight their unique contributions, and finally address their impact on Naaia’s compliance offerings.
The frameworks: Who, what, how and why
ISO/IEC 42001
ISO/IEC 42001 is the world’s first standardized approach to AI management. It is an international standard that specifies the requirements for establishing, implementing, maintaining and continuously improving an Artificial Intelligence Management System (AIMS) within organizations. A management system as defined in ISO/IEC 42001 (3.4), is a “set of interrelated or interacting elements of an organization to establish policies and objectives, as well as processes to achieve those objectives”.
It is therefore designed for entities that supply or use AI-based products or services, to ensure that the development and use of AI systems are responsible, ethical and in line with the organization’s objectives.
Structure
ISO sets out a roadmap of fundamental requirements for a complete AIMS. Its approach comprises several stages:
Context of the Organization: This involves understanding and examining the context, activities and objectives of the organization, including the needs and expectations of interested parties, and setting up an AI management system with a clear, defined scope.
Leadership: This is the organizational structure of the leadership element, which the standard considers to be of the utmost importance, and which focuses on commitment of management and its impact on all interested parties, AI policy and the assignment of roles, responsibilities and authorities within the organization.
Roles, responsibilities and authorities: This involves top management assigning and communicating the responsibilities and authorities necessary to ensure compliance of the AI management system and is accountable to top management.
Planning: The next step is to plan actions to mitigate risks and manage opportunities. It is necessary to plan the assessment of AI-specific risks, their treatment, the assessment of the impact of the AI system, the achievement of AI objectives and changes.
Support: This step details support mechanisms such as resources required by the AI management system, the competences, awareness, communication, and documentation control.
Performance evaluation: It is guided by the audit, performance measurements and monitoring by top management (management review).
Improvement: Continual improvement is achieved through the implementation of audits, change management, the management of nonconformities and corrections, and the collection of user feedback.
Analysis
An important aspect of ISO/IEC 42001 is its adaptability and non-binding nature. The standard is a voluntary certification, which means that it is not a mandatory requirement for organizations. The most defining feature of ISO/IEC 42001 is its emphasis on the organization’s approach to implementing and maintaining an effective AIMS. ISO policies allow for flexibility depending on the scale, nature and context of AI use within an organization, as well as the Organization’s specific AI systems, associated risks and the impact of AI on stakeholders. This adaptability makes the standard applicable to a variety of sectors and contexts, from startups to large enterprises. It is thus a very organization-centric framework.
Overall, ISO/IEC 42001 focuses on internal management practices, helping organizations to meet broader regulatory requirements, making it a valuable tool to adopt and adapt to any regulatory requirements that may arise, including those of the AI Act.
NIST AI Risk Management Framework (RMF)
The NIST AI RMF is a comprehensive guideline developed by the National Institute of Standards and Technology (NIST) of the United States that was published in January 2023. It is a non-sector specific, right-preserving, voluntary framework whose objective is to guide organizations involved in the design, development, deployment and use of AI systems to manage the risks related to AI and promote their trustworthy use and development.
The NIST AI RMF emphasizes both the mitigation of negative impacts and harms to people, organizations, and ecosystems from AI systems. By offering structured approaches to manage potential harms, the framework aims to foster more trustworthy AI technologies and the maximization of positive outcomes from AI systems.
The standard also defines the key characteristics of trustworthy AI, which include valid and reliable, safe, secure and resilient, accountable and transparent, explainable and interpretable, privacy-enhanced, and fair with harmful bias managed. These guidelines provide a robust foundation for developing AI technologies that are ethical, reliable, and beneficial to society at large.
Structure
The framework is structured around four key functions: GOVERN, MAP, MEASURE and MANAGE. Each function is then divided into categories and sub-categories with suggested actions:
GOVERN: This function aims to foster a culture of risk management in AI, providing processes to identify, assess and manage risk while aligning the design of AI systems with the Organization’s values and principles throughout the product lifecycle.
MAP: This function aims to establish context and identify AI-related risks by improving the ability to assess and understand AI systems. It includes checking assumptions, recognizing when systems are operating outside their intended use, identifying both positive applications and limitations, and anticipating risks, including potential negative impacts from both intended and unintended uses.
MEASURE: This function uses tools to assess and monitor AI-related risks identified in the MAP function, tracking metrics relating to trustworthy AI and social impact through rigorous testing and independent review. Its findings inform the MANAGE function for effective risk monitoring and response.
MANAGE: This function allocates resources to address AI-related risks, mapped and measured using the practices established within the GOVERN function. It includes planning for response, recovery, and communication regarding incidents or events.
The standard provides suggested actions however, they are not meant to serve as a checklist or a sequence of steps, they can be summarized as follows: it starts with the establishment of governance structures, followed by mapping the context, then measuring the risks and finally managing the risks. Although the standard can be used to determine risk priorities, it does not define specific risk tolerances.
Analysis
The NIST AI RMF takes a comprehensive and robust approach to AI risk management, addressing technical, ethical, legal and societal factors. Its regulation and law agnostic structure allow organizations in all sectors to tailor the framework to their specific needs and can serve as a complement to other relevant regulatory frameworks with which an organization must comply. It enables organizations to understand how to manage risks throughout the AI system lifecycle, and enables continuous monitoring and adaptation, recognizing that AI-related risks evolve over time, including unanticipated ones.
It also defines insights about the challenges of AI risk management such as risk measurement, including those coming from third parties, identifying a risk tolerance, which the NIST does not prescribe, risk prioritization, and risk integration and management.
By aligning with the NIST AI RMF, organizations can enhance their processes for governing, mapping, measuring, and managing AI risks, while improving the documentation of outcomes. This alignment fosters greater awareness of trustworthiness trade-offs and AI risks, supports clear decision-making for system deployment, and strengthens organizational accountability and culture around AI risk management. Additionally, it encourages better information sharing, a deeper understanding of downstream risks, stronger stakeholder engagement, and improved capacity for test, evaluation, validation, and verification (TEVV) of AI systems.
Although developed in the USA, the NIST AI RMF is a globally recognized standard and considered a valuable guide to meeting international AI standards. Its alignment with global regulations makes it useful for Organizations worldwide to build trustworthy and compliant AI systems.
Certification of the LNE process for AI: design, development, evaluation and maintenance in operational conditions
Process Certification for AI: Design, Development, Evaluation and Maintenance in Operational Conditions, is a certification designed by the Laboratoire National d’Essais (LNE), a leading testing and evaluation organization in France, in 2021, aimed at helping companies engaged in the development, supply or use of AI systems, to integrate, adopt and systematize best practices throughout the AI lifecycle. By providing clear, objective-based references, the LNE certification helps developers adhere to solid technical benchmarks and enables users to confidently adopt AI solutions.
The certification is highly process-oriented, defining specific technical requirements for each phase of the AI lifecycle. It is also sector-independent, meaning that it is eligible for all industries. Its scope encompasses all processes related to AI systems based on machine learning, including static, incremental and hybrid AI. However, it excludes purely symbolic AI systems.
Structure
The LNE certification structure is aligned with the lifecycle of artificial intelligence systems, establishing clear processes for each phase:
Design phase: This phase focuses on transforming user requirements into functional specifications, covering all subsequent choices, from development and quality assurance to communication. During this phase, design features are aligned and checked against normative and regulatory requirements.
Development phase: This phase implements the designed processes through technical development choices, including data management, development procedures, traceability mechanisms, and infrastructure choices, to create a version of the AI system ready for clear evaluation and continuous improvement. The LNE framework is very technically detailed in terms of data management and traceability requirements.
Evaluation phase: This phase validates whether the AI system meets the specifications defined prior to deployment, by means of a comprehensive and structured evaluation protocol, including performance, quality, safety and transparency test procedures.
Operational maintenance phase: After deployment, the AI system is closely monitored under operational conditions to ensure its post-deployment reliability, safety and compliance. This monitoring focuses on potential degradations in performance and compliance throughout the system’s lifecycle, using specific mechanisms to control and correct them. For example, characteristics are continuously monitored to maintain optimum operating conditions.
Communication and documentationrequirements serve as fundamental pillars throughout these phases, promoting transparency, information-sharing and continuous improvement.
Analysis
What sets LNE certification apart is its comprehensive, technical focus on processes. The Certification focuses on the functionality of the AI itself, and how its design, implementation, testing and maintenance meet the highest standards of safety, performance and compliance. This organization- and industry-neutral approach facilitates the definition of widely applicable requirements, which can be easily translated into legal/organizational requirements, as well as technical references.
Another important aspect of the LNE framework is its alignment with industry regulations and emerging legislation. Despite being primarily process-focused, the LNE framework incorporates risk management principles, defining requirements for risk classification and management, to promote ethical use of AI and supply chain resilience. It also defines compliance requirements in all phases of the AI lifecycle to meet a variety of standards and regulations, and to draw the attention of all professionals working in the corresponding AI phase to legal and ethical principles in a structured manner.
Comparison
Framework typology and binding force
The ISO 42001, NIST AI RMF and LNE are all voluntary frameworks for trustworthy AI. Organizations can also choose to be certified by these standards, with the exception of NIST AI RMF, which is not a certification process. However, once organizations commit to this certification process, they become bound by the obligations of these specific frameworks (ISO, LNE).
A comparative table below summarizes and compares each standard by family of actions, highlighting the main orientations and approaches proposed by each standard.
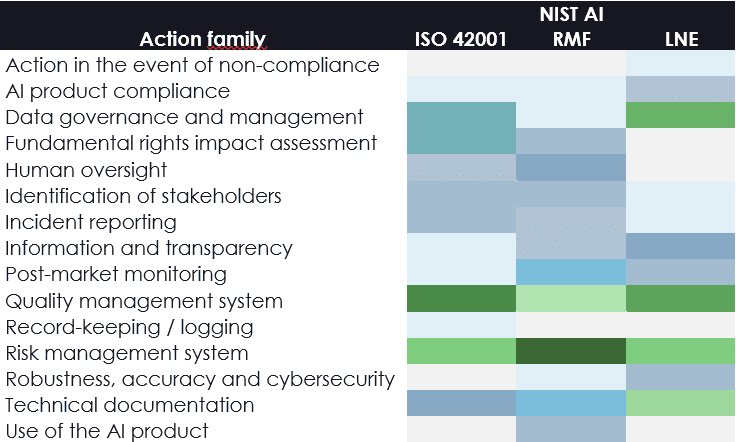
The ISO 42001, NIST AI RMF and LNE are all voluntary frameworks for trustworthy AI. Organizations can also choose to be certified by these standards, with the exception of NIST AI RMF, which is not a certification process. However, once organizations commit to this certification process, they become bound by the obligations of these specific frameworks (ISO, LNE).
Main approaches
ISO 42001 focuses on a comprehensive organization-wide AI management framework, offering a holistic approach to AI systems management. It emphasizes a broad governance framework and the integration of best practices throughout the lifecycle of AIsystems; the NIST AI RMF, meanwhile, focuses more on a risk management framework that emphasizes a structured approach to risk identification, measurement and mitigation, with a focus on ensuring the reliability of AI systems ; lastly, the LNE is more specific to the processes involved in AI development and lifecycle management, with a strong emphasis on ethics, reliability and solution quality. It is therefore a process-based technical framework.
Main objectives
ISO 42001 provides a solid framework for the responsible development, deployment and use of AI systems. It aims to ensure compliance with regulations (European AI Act), continuously improve internal processes, and guarantee safety, fairness, transparency and traceability through policies tailored to the organization.
The NIST AI RMF provides a comprehensive framework for AI risk management throughout the lifecycle of AI systems, with the main objective of fostering trustworthiness by addressing issues such as fairness, transparency, accountability and security. The framework focuses on the continuous improvement of AI governance and risk management practices. By providing a structured approach to mapping, measuring and managing or mitigating AI-related risks, the NIST AI RMF helps organizations develop responsible and trustworthy AI systems. It encourages broad stakeholder engagement and the integration of diverse perspectives, including feedback, making it a versatile tool for achieving effective and ethical AI management on a global scale.
LNE seeks to certify the quality of processes and demonstrate control over the lifecycle of AI systems. This certification guarantees that AI solutions meet security, privacy, compliance and performance requirements, with particular attention paid to avoiding bias and ensuring system robustness. Unlike ISO and NIST, the LNE defines balanced requirements for the design, development, evaluation and maintenance/monitoring of AI systems. In this respect, it is more technical than the other two frameworks, elaborating specific requirements that go beyond risk and quality management.
Target audience and benefits
ISO 42001 is aimed at companies seeking to structure and manage their AI systems on an organization-wide scale, promoting a dynamic of continuous improvement. It aims to strengthen the confidence of stakeholders (internal and external) through strong governance. Its main advantage lies precisely in this organizational and quality management approach, which provides a roadmap adaptable to the organization’s objectives and size. ISO/IEC 42001 is an internationally recognized standard that provides a global framework for responsible AI governance, offering broader credibility and reproducibility than the region-specific NIST and LNE frameworks, which are tailored to the US and EU markets respectively.
The NIST AI RMF is primarily aimed at a wide range of AIactors and organizations involved in the AI lifecycle, including the design, development, deployment and evaluation, use and those involved in the management of the risks of AI systems. Its main distinction from ISO and LNE is that it places greater emphasis on the risk management system aspect of AI systems than the other two standards, which focus more on the quality management system aspect.
LNE certification is aimed at AI developers, suppliers and integrators who wish to demonstrate their mastery of the entire AI lifecycle and an increased level of technical refinement, while ensuring performance, compliance, and ethical standards. The main difference in relation to the NIST and ISO frameworks is the focus on the AI functionality itself, and the careful technical approach to all processes related to AI development, management and deployment.
Relationship with the AI Act
The NIST AI RMF, ISO/IEC 42001 and LNE are all voluntary frameworks, which means that, unlike the AI Act, organizations choose to align themselves with these frameworks, without any binding obligations. Organizations can also choose to be ISO/IEC 42001 and LNE certified (unlike the NIST AI RMF).
These three standards are also intended to complement the AI Act and other regulations, as they all emphasize the need for robust processes and policies as tools for improving compliance. They also share a similar approach to quality and risk management, such as the importance of compliance assessments, improving interoperability and ensuring reliable and responsible use of AI.
ISO/IEC 42001 and LNE certifications both make a valuable contribution to an organization’s AI governance framework, complementing the AI Act. ISO 42001 offers a holistic organizational approach, while LNE focuses on the technical and procedural aspects of AI functionality, enhancing overall AI governance and safety. The NIST AI RMF goes even further, addressing AI-related risks in all systems, including those not classified as high-risk by the AI Act.
All three frameworks – ISO 42001, LNE and NIST AI RMF – can work synergistically with the AI Act and other relevant AI regulations. They emphasize the importance of robust processes and policies, compliance assessments and evaluations, enhancing their interoperability and promoting effective compliance and risk management.
Conclusion
By integrating these complementary frameworks, Naaia strengthens its ability to offer customized compliance solutions. By positioning itself as a key player, Naaia offers strategic guidance on three essential standards: ISO 42001, NIST AI RMF and LNE. This enables each organization to choose, if it so wishes, the standard best suited to its culture, activities and long-term objectives, giving it the opportunity to find the model that suits it best.